Ross Dobson
I look forward to employing my skills in non-academic contexts through the CDT industrial placements.
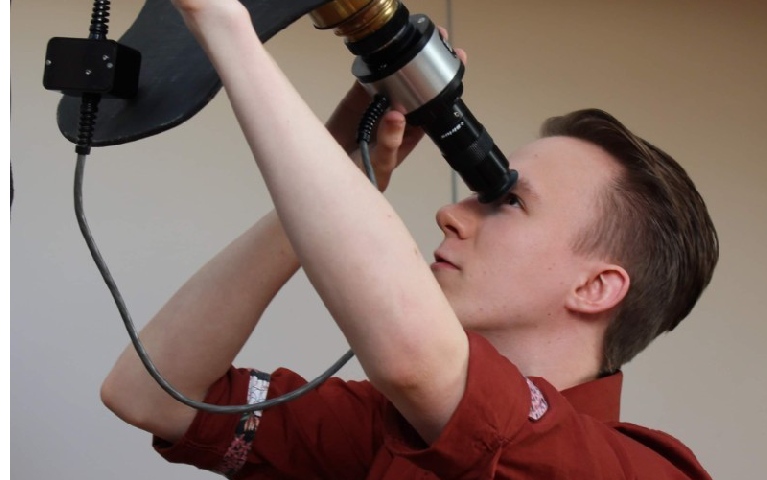
1 February 2021
Project title: Unveiling the exoplanet population with novel data science techniques
Research Group: Astrophysics
Supervisor(s): Dr Vincent van Eylen
Introduction:
I completed my undergraduate studies at UCL, receiving an MSci in Astrophysics in 2021. My 3rd year group project focused on "hot Jupiter" exoplanets, while my MSci dissertation was on the calibration of astronomical instrumentation. I also completed an internship in machine learning at the Mullard Space Science Laboratory, using recurrent neural networks to predict extreme space-weather events and the risks posed to satellite technology, electrical grids and telecommunication networks.
These experiences in data-intensive science drove me to join the CDT: as we enter an increasingly interdisciplinary world, data analysis and artificial intelligence skills are crucial for addressing future scientific and industrial challenges. The DIS CDT offers a unique and exciting opportunity to further develop my skills and apply them in non-academic contexts through the industrial placements.
Outside of my research, I am a strong advocate of science outreach: I help teach coding to school students from disadvantaged and under-represented backgrounds, and I run public tours and work as a teaching assistant at UCL Observatory.
Project description:
The wealth of new data from recent space missions such as Gaia, Kepler and the Transiting Exoplanet Survey Satellite (TESS) presents an opportunity to uncover new insights about the exoplanet population, and to help answer some key questions: for example, which planets are the most favourable and interesting targets for studies of their atmospheres? How unique is our Solar system – and are we alone in the universe?
During the past few years enormous progress has been made on tackling these questions but many outstanding challenges remain. In particular, the increasing volume and complexity of these novel datasets can make it challenging to fully analyse and understand. The current tool of choice for the detection of new exoplanets is Fourier-like methods making use of exoplanet periodicity, but there is some evidence that Machine Learning methods can detect planets which are otherwise missed. Methods such as Deep Learning would be particularly useful to detect signs of rare but exciting planets, such as the atypical transit shapes of disintegrating planets, or the aperiodic orbits of planets orbiting binary stars – planets which have largely eluded systematic searches to date.
However, the goal is not only to detect and classify transiting exoplanets, but also a wide variety of other interesting astronomical phenomena which can be inferred from the rich new datasets, such as detecting binary stars, measuring the rotation rate of stars due to spot modulation, and detecting stellar oscillations which can be used to gain detailed insight into stellar characteristics (known as asteroseismology). This will be achieved through developing Bayesian, Monte Carlo and Machine Learning techniques to combine TESS observations with astrometry data from ESA’s Gaia mission.
First year group project: NCC Group
Placement: